Predictive analytics has rapidly emerged as a powerful tool that allows businesses to make data-driven decisions, especially in the marketing landscape. By leveraging the power of artificial intelligence (AI) and machine learning algorithms, marketers can now forecast customer behavior, preferences, and trends. This invaluable insight helps businesses strategize effectively, anticipate consumer needs, and optimize campaigns for better results. In this article, we will explore the world of predictive analytics using AI and its impact on marketing strategies.
What is Predictive Analytics?
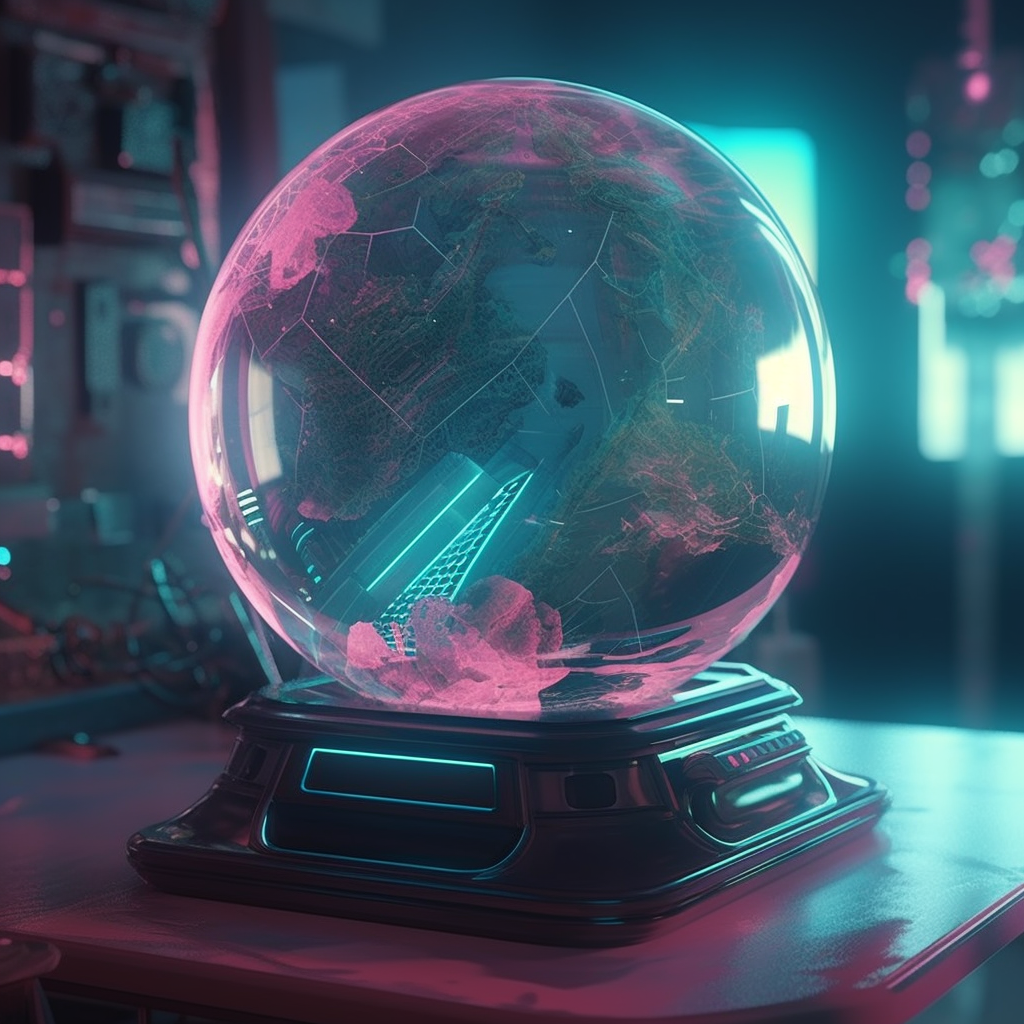
Predictive analytics is a method of using historical data to identify patterns and trends, enabling organizations to make informed predictions about future events. It combines various techniques, including statistical analysis, data mining, and machine learning, to analyze past performance and forecast future outcomes. This approach has gained popularity across industries, as it allows businesses to optimize their resources and make informed decisions.
The Role of Artificial Intelligence in Predictive Analytics
AI plays a critical role in enhancing the capabilities of predictive analytics. The key AI technologies involved are:
3.1 Machine Learning Algorithms
Machine learning is a subset of AI that enables computer systems to learn from data and make predictions or decisions without explicit programming. By employing machine learning algorithms, marketers can analyze vast amounts of data to uncover hidden patterns and relationships that can help predict customer behavior and preferences.
3.2 Deep Learning Techniques
Deep learning is a more advanced form of machine learning that uses artificial neural networks to process data. These networks can automatically discover intricate structures in large datasets, making them highly efficient for tasks like image recognition, natural language processing, and predictive analytics.
Predictive Analytics in Marketing
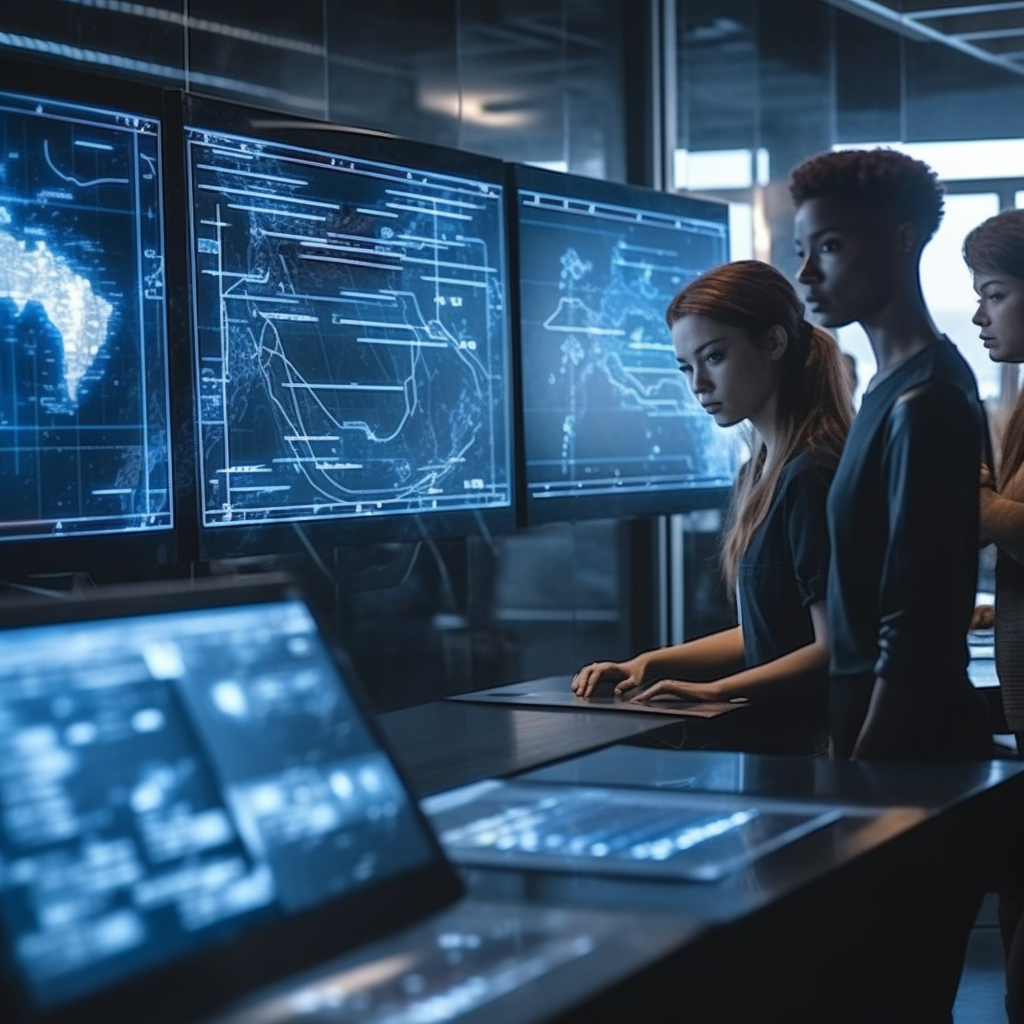
In the marketing domain, predictive analytics has become a game-changer, enabling businesses to anticipate customer needs and make data-driven decisions. It helps marketers identify potential customer segments, optimize campaigns, and allocate resources effectively. This, in turn, leads to improved customer satisfaction, higher retention rates, and increased ROI.
Benefits of Predictive Analytics for Marketers
Predictive analytics offers several advantages for marketers, including:
5.1 Forecasting Customer Behavior
By analyzing historical data, marketers can predict how customers are likely to behave in the future. This can help them tailor marketing messages, offers, and incentives to target specific customer segments and drive desired actions.
5.2 Identifying Customer Preferences
Predictive analytics can help identify individual customer preferences, such as preferred communication channels, product preferences, and purchasing habits. This information enables businesses to personalize marketing campaigns, resulting in higher conversion rates and better customer satisfaction.
5.3 Recognizing Market Trends
By staying ahead of market trends, businesses can capitalize on new opportunities and stay ahead of competitors.
[Continue writing please]
5.4 Enhancing Customer Lifetime Value (CLV)
Predictive analytics can help marketers estimate the CLV of individual customers. By understanding the potential value of customers over time, businesses can prioritize their marketing efforts and allocate resources more effectively to retain high-value customers.
How Businesses Can Leverage Predictive Analytics
To make the most of predictive analytics in marketing, businesses should:
- Collect and integrate relevant data from various sources, such as CRM systems, social media, and customer feedback.
- Invest in advanced AI and machine learning tools to process and analyze the data.
- Establish clear objectives and KPIs for marketing campaigns, informed by predictive insights.
- Continuously monitor and refine marketing strategies based on real-time data and predictive analytics outputs.
Key Components of an Effective Predictive Analytics System
An effective predictive analytics system should include:
- A comprehensive data repository that consolidates relevant data from various sources.
- Robust data cleaning and preprocessing techniques to ensure data quality and accuracy.
- Advanced AI and machine learning algorithms capable of handling large volumes of data.
- An intuitive dashboard that provides actionable insights and recommendations for marketing professionals.
- Integration with existing marketing platforms and tools for seamless execution of data-driven strategies.
Real-World Examples of Predictive Analytics in Marketing
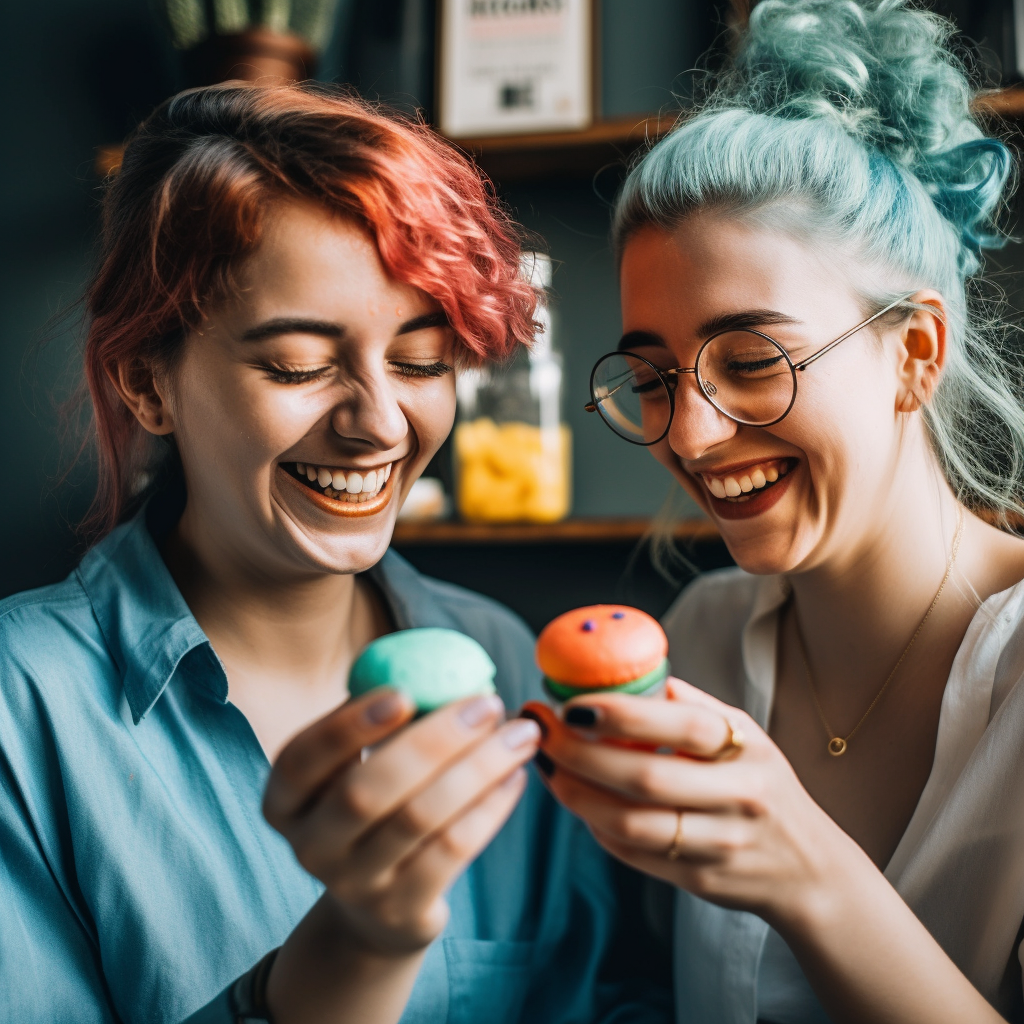
Several companies have successfully implemented predictive analytics in their marketing strategies:
- Amazon uses predictive analytics to recommend products to customers based on their browsing history, purchase patterns, and preferences.
- Netflix leverages machine learning algorithms to analyze user behavior and offer personalized content recommendations, resulting in increased viewer engagement and satisfaction.
- Starbucks employs predictive analytics to optimize its store locations, menu offerings, and promotional strategies, driving customer loyalty and sales growth.
Challenges in Implementing Predictive Analytics
Despite its numerous benefits, implementing predictive analytics in marketing comes with its share of challenges, such as:
- Data quality and consistency: Ensuring accurate and reliable data is crucial for generating meaningful insights.
- Data security and privacy: Protecting customer data and complying with privacy regulations is paramount.
- Skillset and expertise: Developing and maintaining a predictive analytics system requires specialized skills and knowledge in AI, machine learning, and data science.
The Future of Predictive Analytics and AI in Marketing
As AI and machine learning technologies continue to advance, predictive analytics will become even more integral to marketing strategies. Marketers can expect increased accuracy and sophistication in their predictions, enabling them to deliver more personalized and relevant experiences to customers. Additionally, the integration of AI-powered predictive analytics with other emerging technologies, such as virtual reality and the Internet of Things, will open up new opportunities for innovation and growth in the marketing landscape.
Conclusion
Predictive analytics using AI has transformed the marketing landscape by enabling businesses to forecast customer behavior, preferences, and trends. By leveraging advanced machine learning algorithms, marketers can optimize their campaigns, anticipate consumer needs, and stay ahead of the competition. As AI technologies continue to evolve, businesses must invest in predictive analytics to remain competitive and drive marketing success.
Frequently Asked Questions (FAQs)
- What is the difference between predictive analytics and traditional analytics?
Predictive analytics focuses on forecasting future events based on historical data, while traditional analytics involves analyzing past data to understand what has already occurred. - How does AI enhance predictive analytics?
AI, through machine learning and deep learning algorithms, helps uncover hidden patterns and relationships in large datasets, improving the accuracy and effectiveness of predictions. - What industries can benefit from predictive analytics?
Predictive analytics can benefit various industries, including marketing, finance, healthcare, manufacturing, and retail, among others. - **What are the key
[Continue writing please]
components of an effective predictive analytics system?**
An effective predictive analytics system should have a comprehensive data repository, robust data cleaning and preprocessing techniques, advanced AI and machine learning algorithms, an intuitive dashboard, and seamless integration with existing marketing platforms and tools.
- What are some potential challenges in implementing predictive analytics?
Some challenges in implementing predictive analytics include ensuring data quality and consistency, addressing data security and privacy concerns, and acquiring the necessary skillset and expertise to develop and maintain the system.
[Please list 5 AI tools that help with the functions described above]
Here are 5 AI tools that can assist with predictive analytics and other functions described in the article:
- Google Analytics Intelligence: A powerful AI-driven analytics tool that provides insights and recommendations for optimizing marketing strategies, forecasting trends, and understanding customer behavior.
- IBM Watson: An advanced AI platform that offers various machine learning and deep learning capabilities to help businesses create predictive models, analyze customer data, and make informed marketing decisions.
- SAS Viya: A cloud-based analytics platform that leverages AI and machine learning to help businesses forecast customer behavior, optimize marketing campaigns, and identify market trends.
- DataRobot: An automated machine learning platform that enables businesses to build and deploy predictive models quickly, helping them make data-driven decisions in marketing and other domains.
- RapidMiner: A data science platform that offers various machine learning and AI tools to help marketers analyze customer data, build predictive models, and optimize marketing strategies.